3D Part Segmentation via Geometric Aggregation of 2D Visual Features
WACV 2025


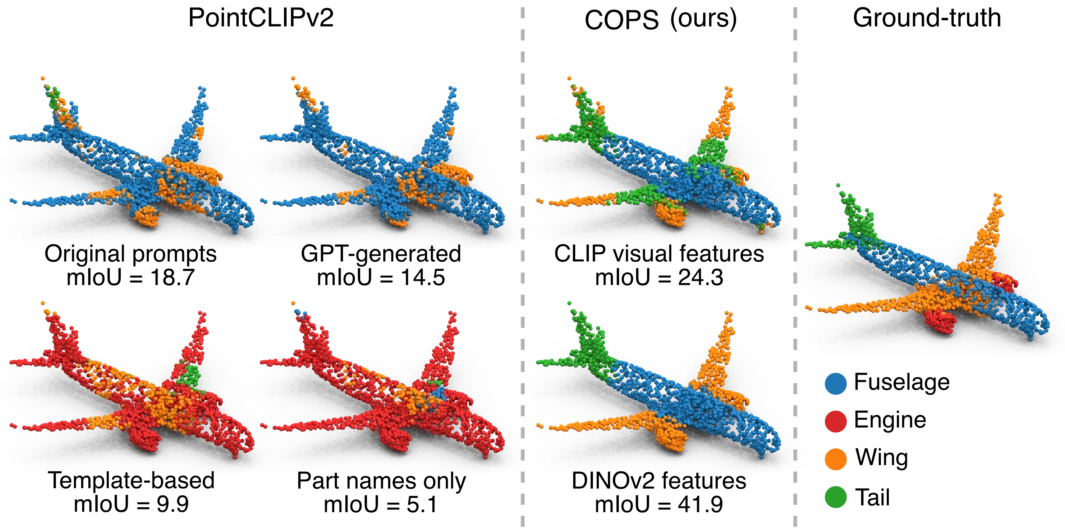
The quality of the parts' description heavily influences the part segmentation performance of methods based on vision-language models. For instance, the performance of PointCLIPv2 (left), deteriorates rapidly when replacing the default textual prompt with e.g., a GPT-generated description, the template "This is a depth image of an airplane's (part)", or simply using part names. In contrast, our pipeline (center) produces more accurate segmentations by disentangling part decomposition from part classification. The improvement is evident when utilising the same CLIP visual features as PointCLIPv2 (top) and further increases when using DINOv2 features (bottom), the default choice of COPS. COPS generates more uniform segments with sharper boundaries, resulting in higher segmentation quality.
ABSTRACT
Supervised 3D part segmentation models are tailored for a fixed set of objects and parts, limiting their transferability to open-set, real-world scenarios. Recent works have explored vision-language models (VLMs) as a promising alternative, using multi-view rendering and textual prompting to identify object parts.
However, naively applying VLMs in this context introduces several drawbacks, such as the need for meticulous prompt engineering, and fails to leverage the 3D geometric structure of objects. To address these limitations, we propose COPS, a COmprehensive model for Parts Segmentation that blends the semantics extracted from visual concepts and 3D geometry to effectively identify object parts. COPS renders a point cloud from multiple viewpoints, extracts 2D features, projects them back to 3D, and uses a novel geometric-aware feature aggregation procedure to ensure spatial and semantic consistency.
Finally, it clusters points into parts and labels them. We demonstrate that COPS is efficient, scalable, and achieves zero-shot state-of-the-art performance across five datasets, covering synthetic and real-world data, texture-less and coloured objects, as well as rigid and non-rigid shapes.
In summary, our contributions are:
• A novel training-free method for 3D part segmentation, which disentangles part segmentation from semantic labelling;
• A geometric feature aggregation module that fuses 3D structural information with semantic knowledge from VFMs;
• A thorough evaluation on five benchmarks, establishing a unified evaluation that future zero-shot part segmentation methods can use for comparison.
• A novel training-free method for 3D part segmentation, which disentangles part segmentation from semantic labelling;
• A geometric feature aggregation module that fuses 3D structural information with semantic knowledge from VFMs;
• A thorough evaluation on five benchmarks, establishing a unified evaluation that future zero-shot part segmentation methods can use for comparison.
We provide additional details and ablations in the supplementary material. You can find them at the end of the arXiv paper.
• Marco Garosi (DISI, University of Trento)
• Riccardo Tedoldi (DISI, University of Trento)
• Davide Boscaini (TeV, Fondazione Bruno Kessler)
• Massimiliano Mancini (DISI, University of Trento)
• Nicu Sebe (DISI, University of Trento)
• Fabio Poiesi (TeV, Fondazione Bruno Kessler)
• Riccardo Tedoldi (DISI, University of Trento)
• Davide Boscaini (TeV, Fondazione Bruno Kessler)
• Massimiliano Mancini (DISI, University of Trento)
• Nicu Sebe (DISI, University of Trento)
• Fabio Poiesi (TeV, Fondazione Bruno Kessler)
You can cite this work as:
@article{garosi2025cops,
author = {Garosi, Marco and Tedoldi, Riccardo and Boscaini, Davide and Mancini, Massimiliano and Sebe, Nicu and Poiesi, Fabio},
title = {3D Part Segmentation via Geometric Aggregation of 2D Visual Features},
journal = {IEEE/CVF Winter Conference on Applications of Computer Vision (WACV)},
year= {2025},
}